RESEARCH PROJECTS (Served as PI)

Light-weighted DNN for Object Detection/Tracking, Body Action Detection and Person Identification
05/01/2017-03/18/2019
(Private Company) PI: Dong Huang, RI, CMU
Modern quadcopters extensively extend the view-angles, range and flexibility of visual perception that were not possible for stationary surveillance cameras. The unique maneuver and hangover capability of quadcopters has presented them in a vital role in improving public safety, personnel safety in the presence of heavy machinery, inventory/freight management, building/bridge maintenance and field rescue. However, most state-of-the-art deep neural networks are too heavy for the computation and memory resources that quadcopters can carry. We develop light-weighted deep neural networks that empower quadcopters with advanced Machine Learning (ML) technologies, and develop multiple building blocks for the general perception and analysis solutions.
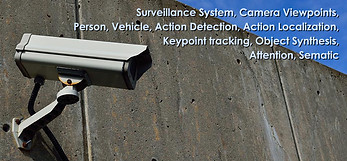
A View-Invariant Internal World Representation for Predictive Cognitive Human Activity Understanding
01/2018-Present
IARPA (Federal Agency) PI: Alex Hauptmann, LTI, CMU;
Co-PI: Dong Huang, RI, CMU
A view-invariant internal representation of human activities and their context is essential for reasoning both normative and abnormal human behavior in a proper situational context. In the same way that changing the viewing angle of a person performing an activity does not change our interpretation of an activity, a truly autonomous surveillance system maintain an interpretation of the scene irrespective of (invariant to) the viewing angle, making it possible to understand, forecast or simulate possible outcomes of anomalous behaviors in real scenarios. In a joint effort with multiple groups at CMU, we develop a portfolio of methods and tools for human activity analysis that makes use of a rich view-invariant internal world representation to detect simple and complex human activities in a video surveillance scenario. My group focuses on efficient activity classification and localization for our autonomous surveillance system.

Affective state estimation from wearable sensors: Phase 0
01/27/2017-03/31/2017
Sony Corporation (Private Company) PI: Dong Huang, RI, CMU
Understanding and detecting human affective state is the vital step for improving quality of life and personalized product services. This project aims to develop core technologies of advanced affective state estimation (e.g. stress, concentration, joy etc.) by vital sensors (e.g. ECG/HR, GSR, BP, EEG). The CMU team will integrate cutting-edge technologies of device, psychology, and machine learning towards advanced affective state estimation.

STTR Phase I: Wearable system for mining Parkinson’s disease symptom states in an
ambulatory setting
01/01/2016-12/30/2017 National Science Foundation co-PI: Britta Ulm, Abililife Inc. co-PI: Dong Huang, RI, CMU
Understanding and detecting human affective state is the vital step for improving quality of life and personalized product services. This project aims to develop core technologies of advanced affective state estimation (e.g. stress, concentration, joy etc.) by vital sensors (e.g. ECG/HR, GSR, BP, EEG). The CMU team will integrate cutting-edge technologies of device, psychology, and machine learning towards advanced affective state estimation.
Face De-Identification for Research and Clinical Use
09/01/2014-08/31/2016 National Institutes of Health PI: Dong Huang, RI, CMU
Recent advances in clinical research requires image and video data of people for either immediate inspection or storage and subsequent analysis. These new trend, however, ignites concerns about the privacy of people identifiable in the scene. In particular, privacy concerns of patients visible and identifiable within the data make this task difficult. These privacy concerns have become the barriers for the widespread use of on videos in health-related behavioral and social science research. To address these concerns, we develop automated methods to de-identify individuals in these videos. Existing methods for de-identification tends to destroy all information involving the individuals by distorting or blurring the face images of the subjects. These methods do not preserve the facial actions of the subjects that contain the valuable information for behavioral and medical studies. In contrast, our advanced face de-identification algorithms are able to obscure the identity of the subject without obscuring the action (i.e. preserving the facial expression that may reveal of a particular medical condition). Our system will be developed by an interdisciplinary team of computer and behavioral scientists, and it will be made available to the medical community.
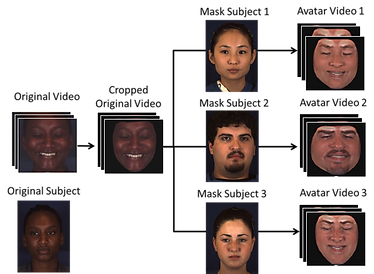